Astronomers Are Using AI to Find Planet-Eating Stars
Astronomers have discovered hundreds of “polluted” white dwarf stars in the Milky Way, thanks to a groundbreaking AI technique. What’s more, these stars are consuming their orbiting planets, providing a unique way to study planetary interiors.
This discovery not only advances our understanding of these stars but also opens new possibilities for exploring the composition of distant planets.
White Dwarfs: Stars in Their Final Stage
White dwarfs are stars that have exhausted their fuel and shed their outer layers. They are slowly cooling remnants of stars like our sun, which will become a white dwarf in about 6 billion years.
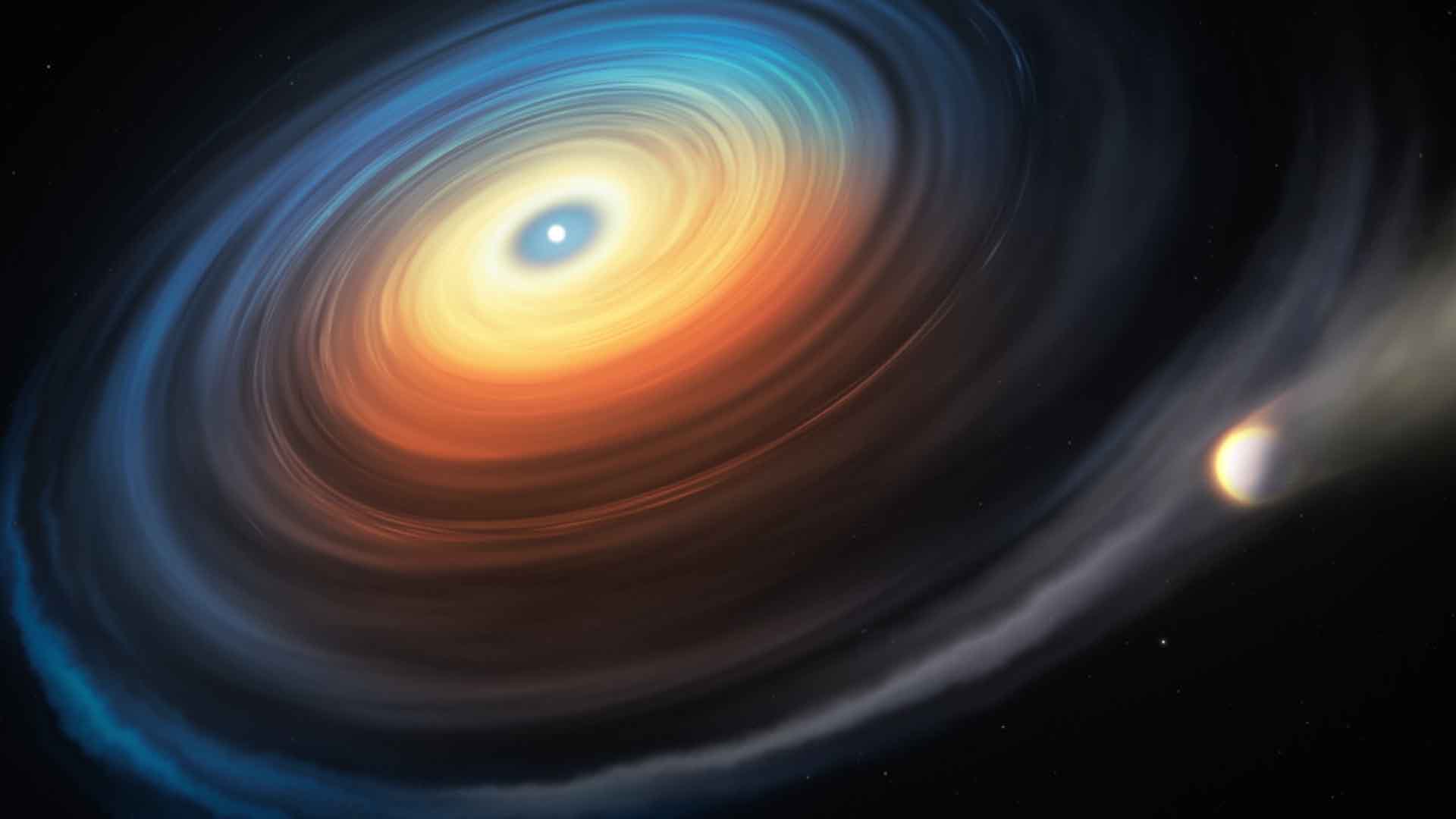
Source: ESO/M. Kornmesser
Studying these stars helps scientists understand the final stages of stellar evolution.
Polluted White Dwarfs
When planets orbiting a white dwarf are pulled in by the star’s gravity, they are ripped apart and consumed.
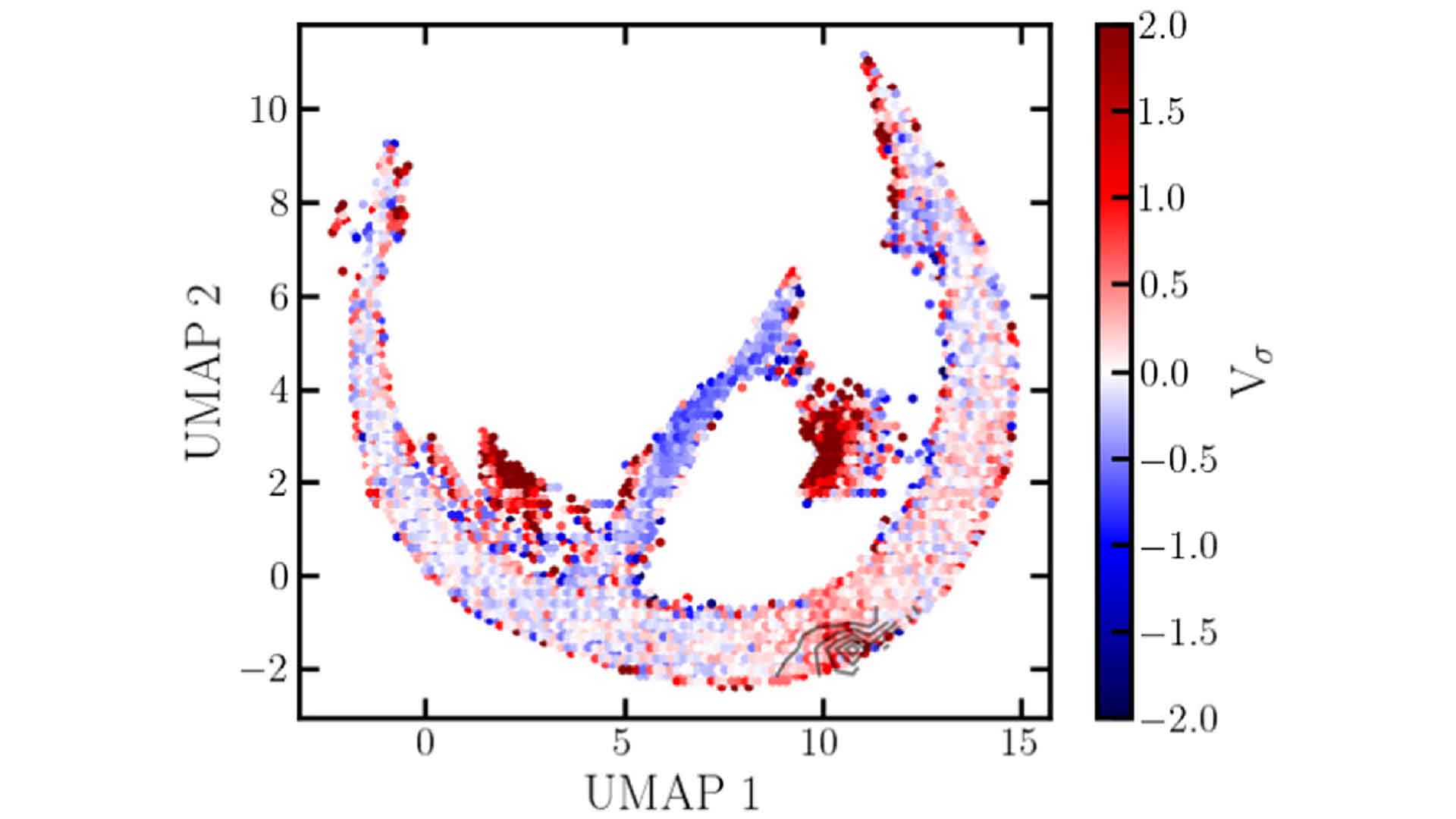
Source: Kao/The Astrophysical Journal
This process “pollutes” the white dwarf with heavy metals from the planet’s interior. These metals, detected in the star’s atmosphere, provide clues about the planets’ composition.
The Challenge of Finding Polluted White Dwarfs
Identifying polluted white dwarfs is challenging because the evidence of heavy metals in their atmospheres is subtle and can be easily missed.
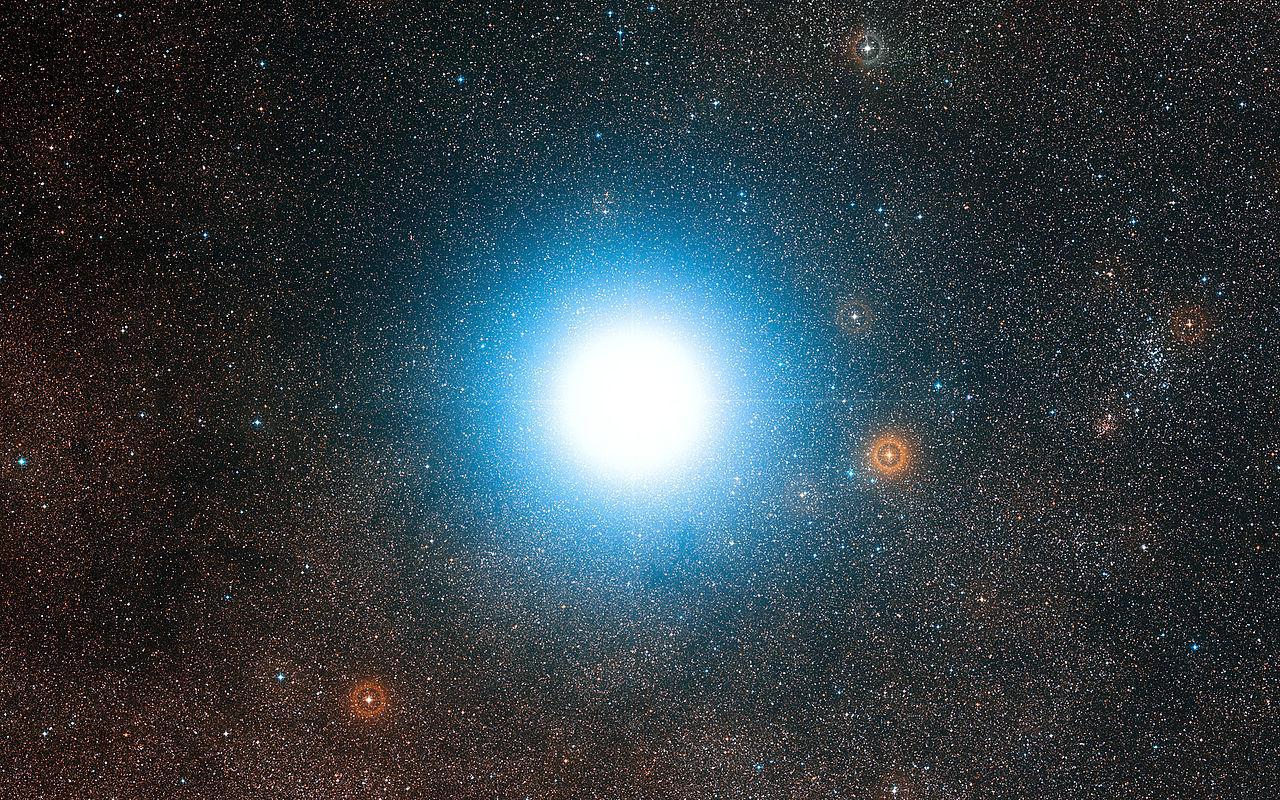
Source: Wikimedia
Traditional methods involve manually reviewing large amounts of survey data, which is time-consuming and labor-intensive.
AI Revolutionizes the Search
A team led by University of Texas at Austin graduate student Malia Kao has developed an AI technique called manifold learning to accelerate the search for polluted white dwarfs.
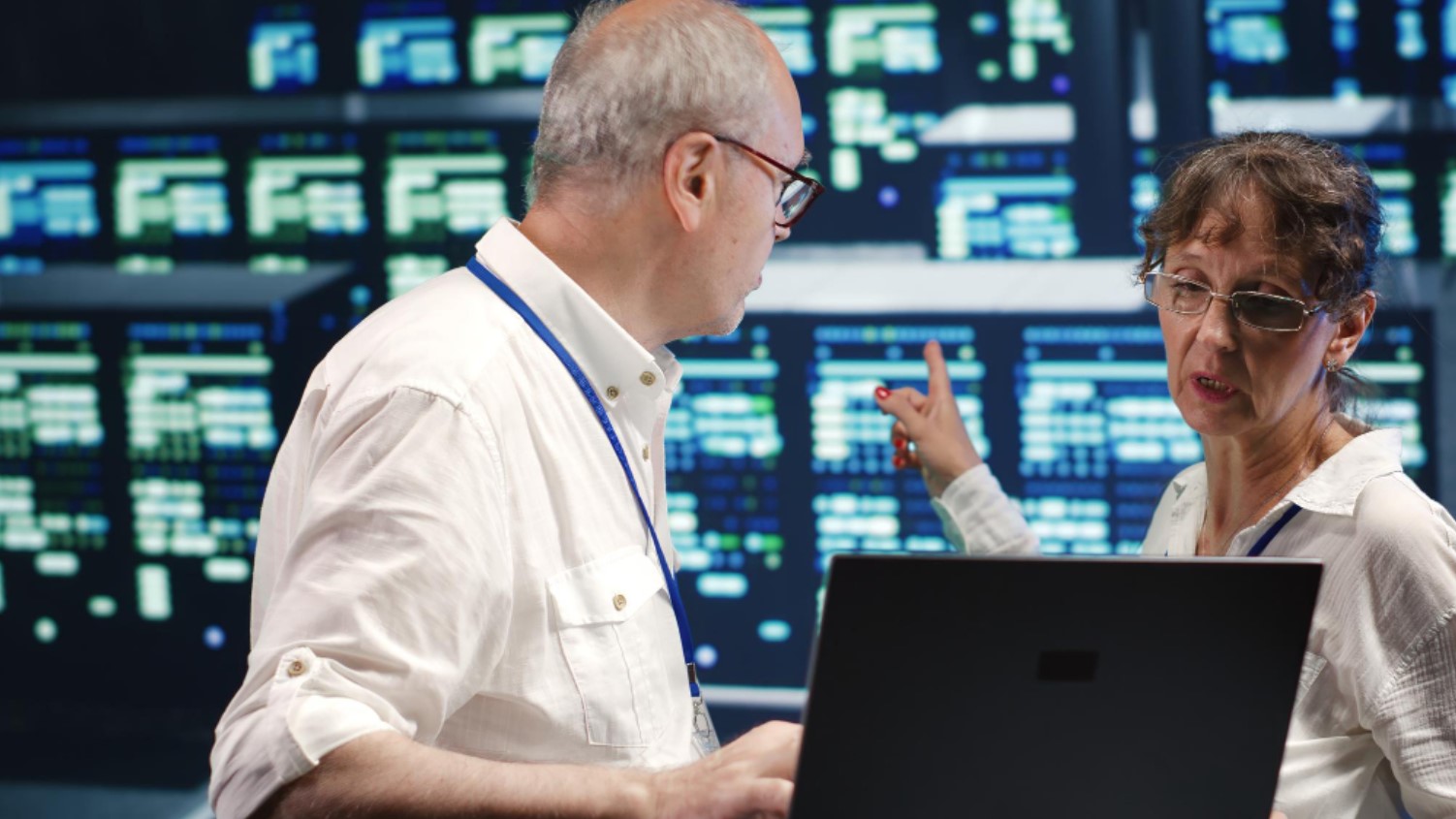
Source: Wikimedia
This method has a 99% success rate in identifying these stars, making the process much more efficient.
How Manifold Learning Works
Manifold learning involves an algorithm that analyzes data and groups similar items together in a visual chart.
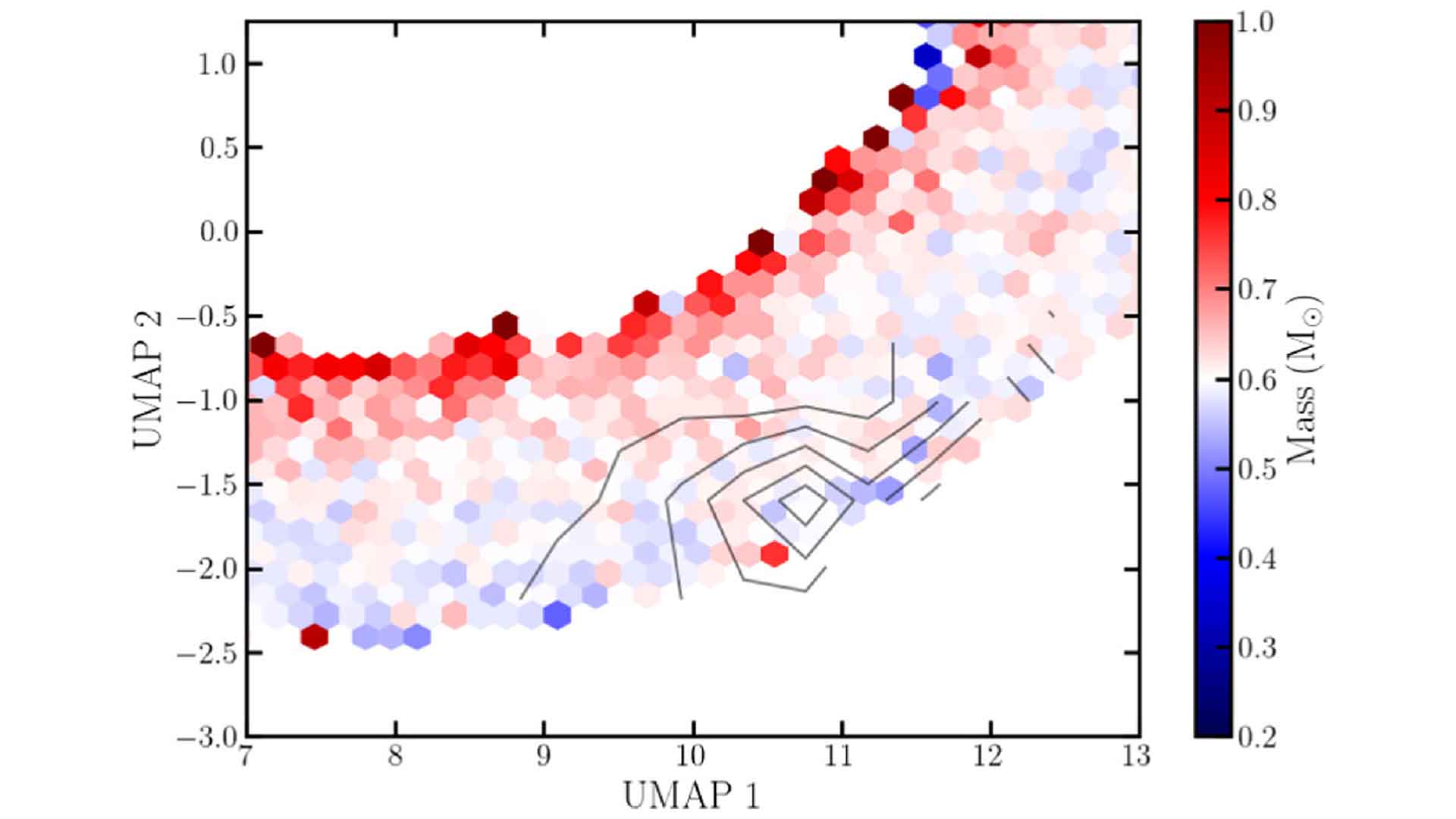
UMAP data
Researchers can then review the chart to identify promising candidates for further investigation, significantly reducing the time needed to find polluted white dwarfs.
Collaborative Efforts and Future Prospects
This research utilized data from the European Space Agency’s Gaia mission and follow-up observations with the Hobby-Eberly Telescope and the Very Large Telescope.
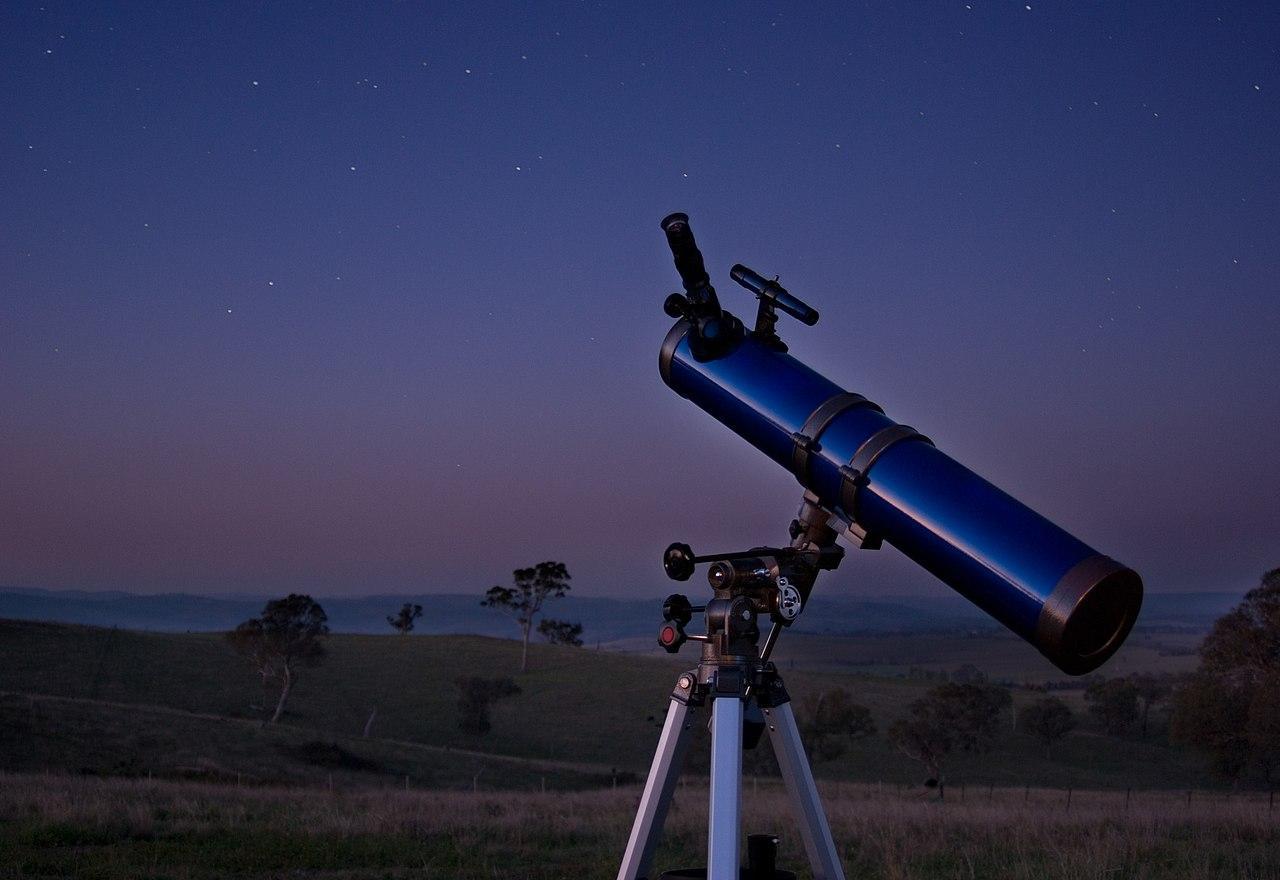
Source: Wikimedia
The Texas Advanced Computing Center provided the necessary resources, demonstrating the importance of collaboration in advancing astronomical research.
Success with Gaia Data
The team used data from the Gaia space telescope, which provides one of the largest spectroscopic surveys of white dwarfs.
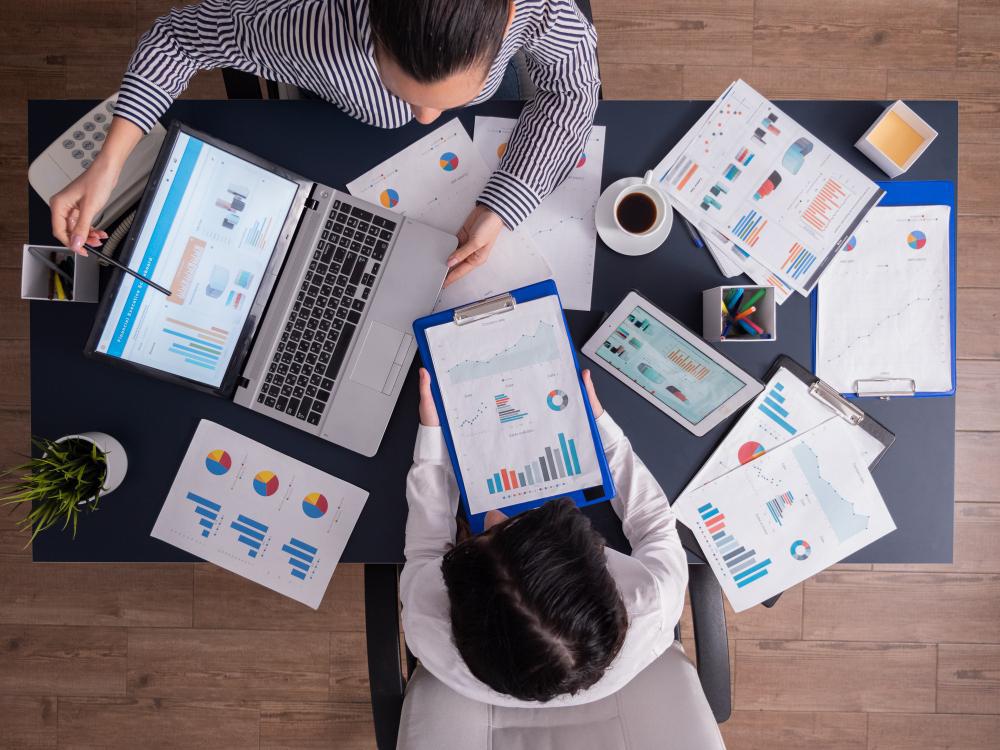
Source: Wikimedia
Although the data is low resolution, the AI technique successfully identified a group of 375 stars showing signs of heavy metal pollution.
Confirming the Findings
Follow-up observations with the Hobby-Eberly Telescope at UT’s McDonald Observatory confirmed the presence of heavy metals in these stars’ atmospheres.
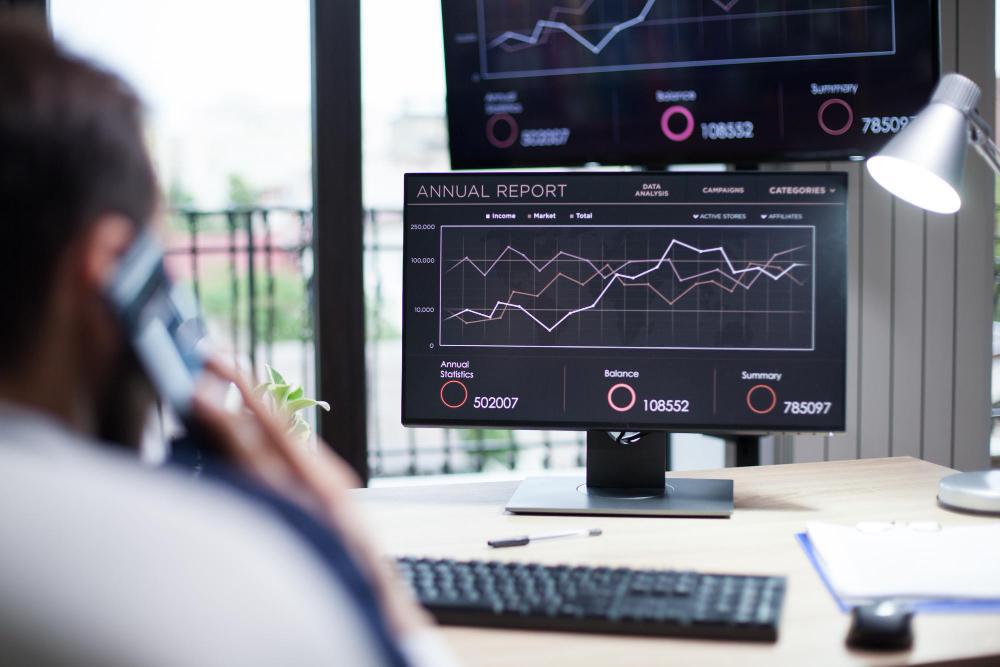
Source: Wikimedia
This confirmation was a crucial step in validating the AI technique’s effectiveness.
A Tenfold Increase in Known Polluted White Dwarfs
“Our method can increase the number of known polluted white dwarfs tenfold,” Kao said.
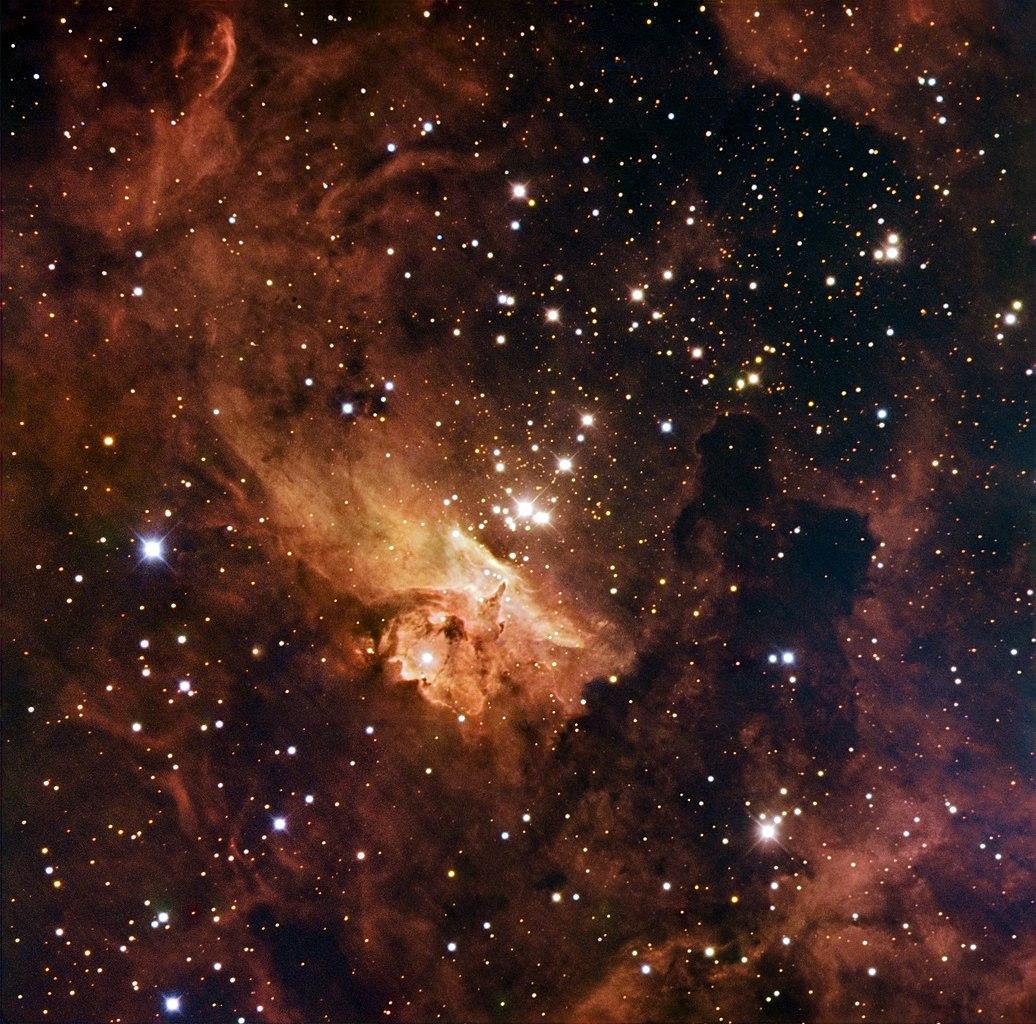
Source: Wikimedia
This increase will allow scientists to better study the diversity and geology of planets outside our solar system, providing valuable insights into planetary formation and evolution.
The Broader Impact of the Research
Keith Hawkins, an astronomer at UT and co-author of the study, emphasized the importance of these findings.
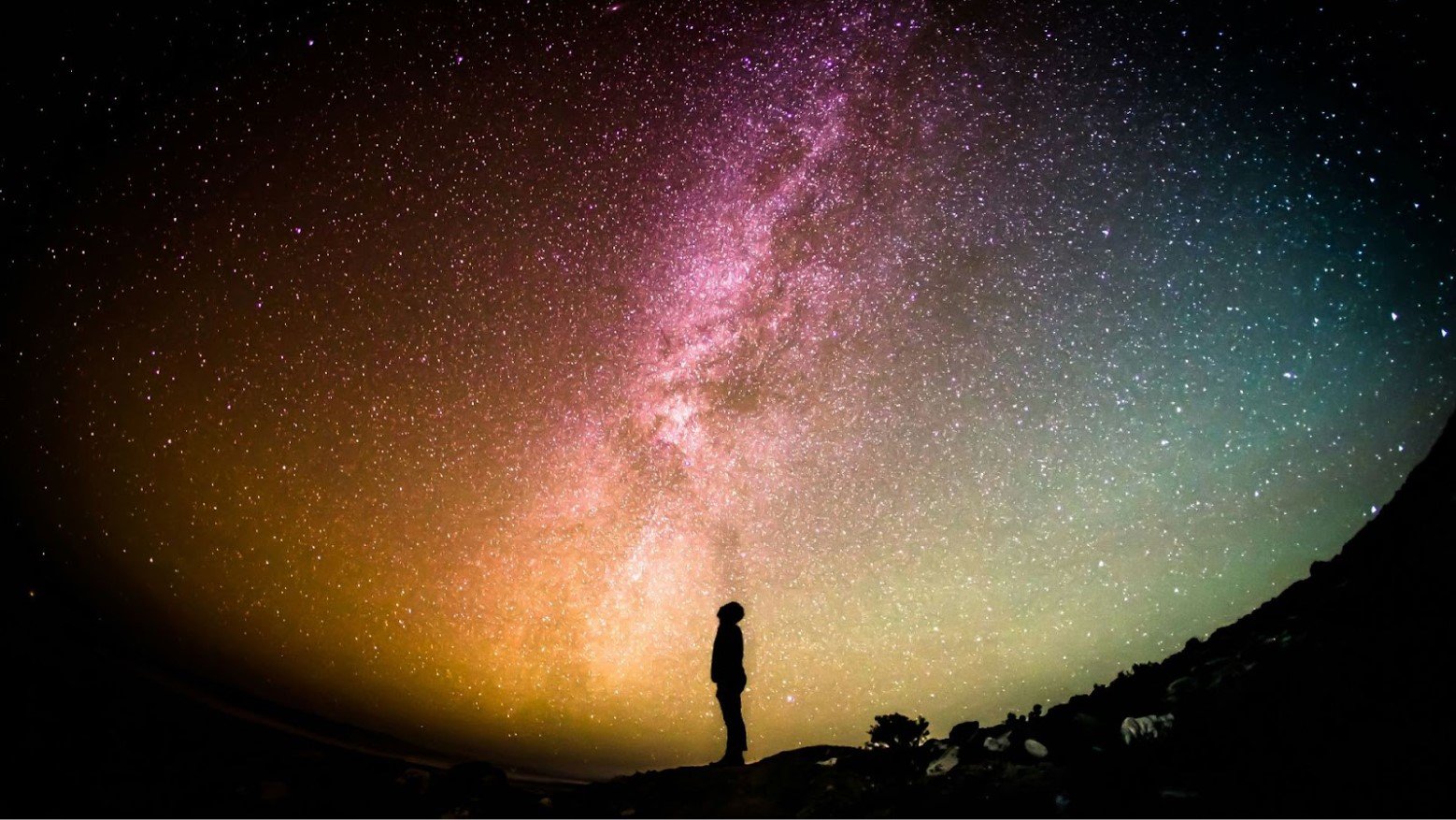
Source: Greg Rakozy/Unsplash
“It’s the only bona fide way to actually figure out what planets outside the solar system are made of,’ Hawkins said.
AI at the Forefront of Astronomical Research
This innovative use of AI is just one example of how researchers at The University of Texas at Austin are solving scientific mysteries.
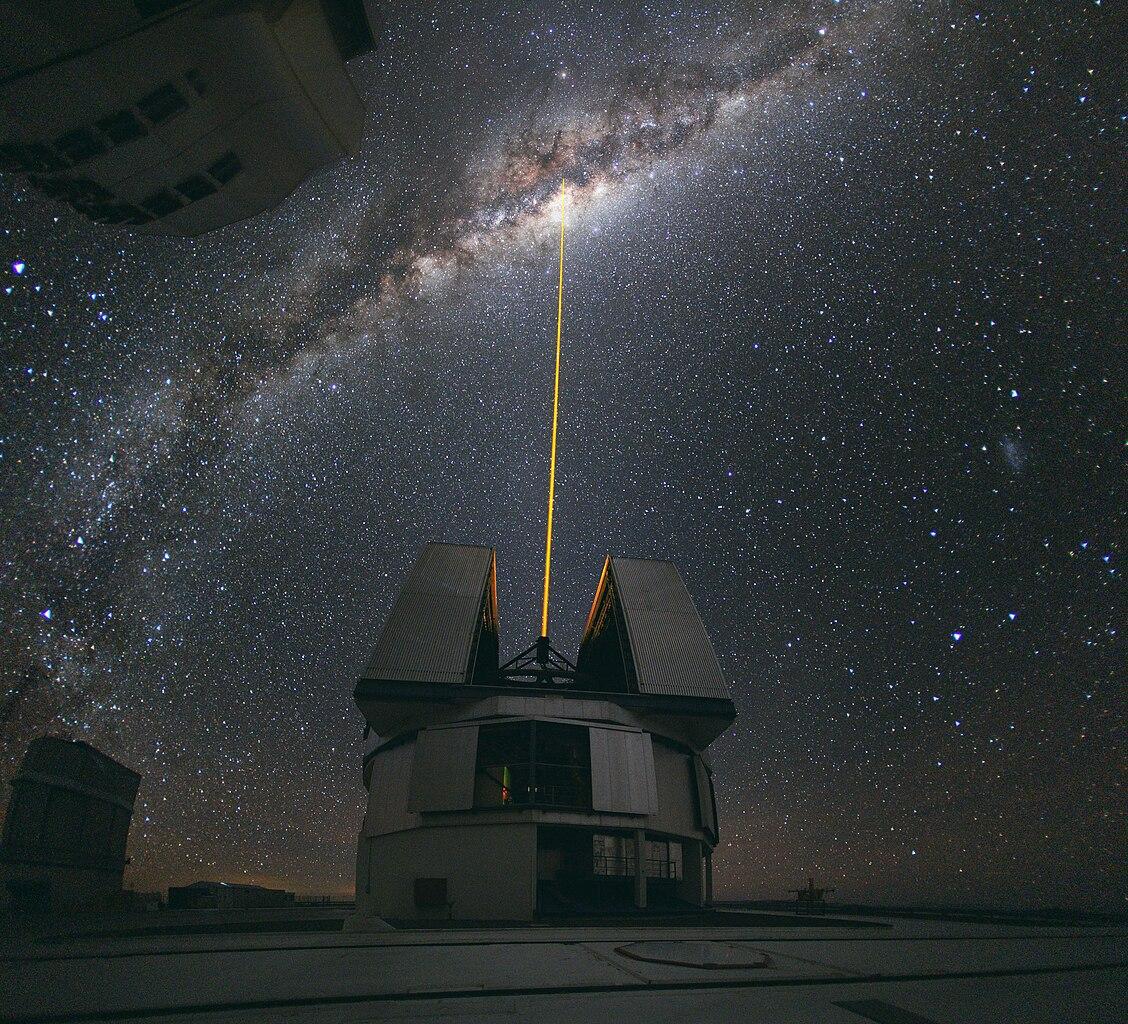
Source: Wikimedia
To highlight these advancements, UT Austin has declared 2024 the “Year of AI,” showcasing the university’s commitment to cutting-edge research.